News Story
Stretchable, soft sensing skins for robots
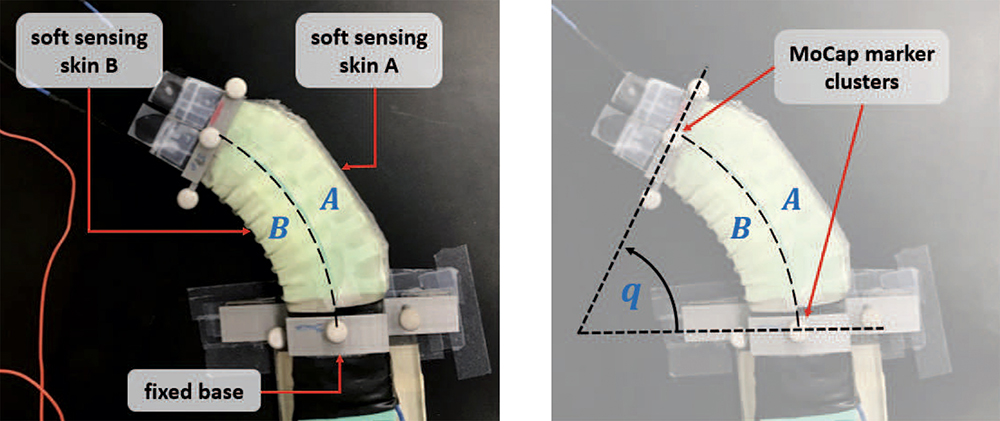
Fig. 2 from the paper shows a soft robot retrofitted with the stretchable sensing skins. The compartments of the soft robot and the sensing skins are labeled in panel (a). Panel (b) shows the degree of curvature and the markers placed for the motion capture (MoCap) system.
Soft robots are made out of low-elasticity materials that can mimic biological materials like skin. The goal of much current research is to create pliant and dexterous soft robots that can manipulate objects in ways similar to, for example, how elephants use their trunks, or octopi use their tentacles. Such soft robots could provide effective manipulation in unstructured environments, from underwater and space operations to minimally invasive surgeries.
Despite considerable recent advances in fabricating, modeling and controlling soft robots, equipping them with integrated sensing and estimation is still in its infancy. This capability could enable a soft robot to perceive the world without relying on external sensors. In particular, creating reliable position awareness (proprioception) in a soft robot is desirable for state estimation and in closed loop control. While several methods have been proposed for developing these sensors, only a few technologies have been demonstrated that would provide closed loop dynamic control for a wide range of soft robots.
In Adaptive Tracking Control of Soft Robots using Integrated Sensing Skin and Recurrent Neural Networks, a team of University of Maryland researchers has developed stretchable, soft sensing skins that operate under the piecewise constant curvature modeling hypothesis. The paper was written by Institute for Systems Research and Mechanical Engineering faculty Elisabeth Smela, Miao Yu and Nikhil Chopra, their students Lasitha Weerakoon and Zepeng Ye, and alum Rahul Subramonian Bama (M.Eng. Robotics 2018).
The skin is a spray-coated piezoresistive sensing layer on a latex membrane that could be retrofitted to many soft robots. Degree of curvature estimation could be learned using a long short-term memory (LSTM) neural network that only requires strain signals from the sensing skin and the actuator inputs.
The researchers also designed an adaptive controller to track a desired degree of curvature trajectory for low and high frequency target trajectories. The research demonstrates that the soft skins can estimate the degree of curvature robustly for inclusion in a dynamic control framework.
Published February 13, 2021